New AI Paradigm Levels the Playing Field for Large Language Model Development
Fusing small domain-specific models into a single powerful one could cut Centralised AI training costs by over 99.9%
In January, China’s DeepSeek artificial intelligence (AI) startup made headlines with the surprise launch of its large language model (LLM). The media frenzy was fuelled by the new offering’s ability to outperform LLMs from the biggest AI tech players, despite having access to less funding and technological resources. However, according to Professor Yang Hongxia of PolyU’s Department of Computing, the most significant aspect of DeepSeek’s LLM is the fact it is entirely open source. Combined with an innovative “Model-over-Models” approach pioneered by her research team, this will enable smaller companies, startups and individual developers to disrupt, enhance, and accelerate LLM development.
Overcoming LLM obstacles
Formerly Head of LLM at ByteDance and AI scientist at Alibaba’s DAMO Academy, Professor Yang believes AI development is being hindered by a de facto monopoly. Training LLMs from scratch requires access to centralised and costly graphic processing unit (GPU) resources, which only a few tech companies can afford. This issue particularly affects enterprise-based generative AI (GenAI), where models trained only on general web data perform poorly in a range of specialised sectors, such as healthcare, material intelligence and energy.
“This gap exists because much of the relevant data for these areas that cannot be crawled from general web hasn’t been incorporated into AI model development,” said Profeesor Yang.
In her opinion, building a comprehensive model that consistently excels across all domains remains challenging.
At a forum organised by The PolyU Academy for Interdisciplinary Research, Professor Yang speaks to over a thousand audiences including leaders from the innovation and technology sector on the topic “DeepSeek and Beyond”.
Model over models
To address the challenge, Professor Yang and her team are leading the development of a “Model-over-Models” (MoM) approach, which builds a foundational model from smaller, stackable domain-specific models.
Called InfiFusion, their solution effectively distils knowledge from diverse source models, regardless their origin or architecture, overcoming vocabulary mismatches and computational inefficiencies.
They also supply continual pretrain platform which opens the door for training AI tailored to specific domains. In fact, it is now possible to combine various domain-specific models into a single model, harnessing their unique advantages without the necessity of retraining a massive, monolithic model.
Experimental data indicates that InfiFusion performs better than other state-of-the-art models, such as Alibaba’s Qwen-2.5-14B-Instruct and Microsoft’s Phi-4, across 11 benchmark tasks, including reasoning, coding, mathematics, and instruction-following. It can also complete trainings at a fraction of the cost – just 0.015% – of traditional centralised methods.
This approach also maximises the utility of less advanced, heterogeneous computing resources, allowing domestic chips to be more effectively used for small model training. This efficiency also positions Hong Kong to lead in GenAI development while fostering China’s AI hardware ecosystem through optimised use of heterogeneous computing resources.
~ Professor Yang Hongxia
Professor Yang is confident that InfiFusion represents an efficient and scalable solution for high-performance LLM deployment. It paves the way to a decentralised LLM, which she views as the future of generative AI.
“We can leverage distributed highperformance computing (HPC) centres equipped with diverse computing accelerators, including those at Cyberport, Science Park, and Zhejiang Lab (Zhejiang HPC Center) via the MoM architecture. Heterogeneous, entrylevel GPUs can be efficiently utilised, in contrast to traditional approaches that require large clusters of identical highend GPUs for training from scratch.”
Leading the AI academy
Professor Yang is now in charge of PolyU’s new PolyU Academy for Artificial Intelligence (PAAI), which was established to drive fundamental scientific breakthroughs and enhance the University’s reputation as an AI leader. Her duties include developing the innovative MoM machine learning paradigm.
PAAI facilitates collaboration among PolyU researchers across diverse disciplines. The goal is to develop AI models with specialised domain expertise, which enable the training of a more general AI model using MoM. The resulting AI models will be suitable for a variety of university applications, including research and teaching, where they could transform language education for students.
Professor Yang and her team are currently developing foundation models in cutting-edge fields, including healthcare, manufacturing, energy, and finance. Their latest research involves working on a cancer foundation model in collaboration with top hospitals in Zhejiang and Beijing.
While current LLMs have made impressive strides in general intelligence, they still fall short in specific domains in fields like manufacturing and biochemistry.
~ Professor Yang Hongxia
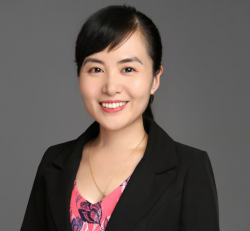
Professor Yang Hongxia | |
• | Associate Dean (Global Engagement), |
• | Professor, Department of Computing |
• | Executive Director, PolyU Academy for |