Professor M.W. Mak
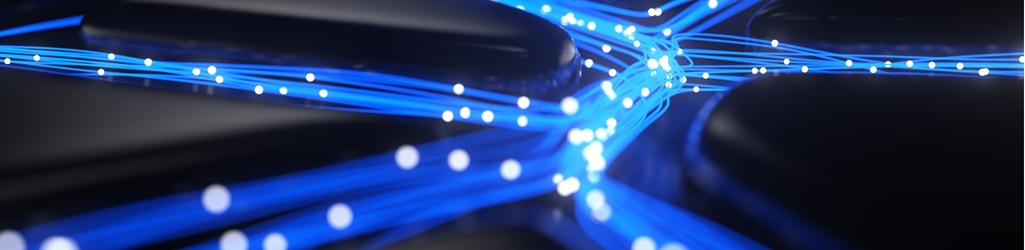
Study
About
Professor Mak has served as an associate editor of IEEE Trans. on Audio, Speech and Language Processing, Journal of Signal Processing Systems, and IEEE Biometric Compendium. He also served as a Technical Committee member of IEEE MLSP. He is a tutorial speaker in Interspeech’2016. Professor Mak’s research interests include speaker recognition, machine learning, and bioinformatics. Professor Mak has been working extensively on machine learning, speaker recognition, and bioinformatics.
Research Highlights
Speaker Recognition: Professor Mak’s recent work in multi-stage meta-learning enables a text-independent speaker verification system to be adapted to a text-dependent one using a few target-phrase utterances only, dramatically reducing the resources required to build text-dependent speaker verification systems. He published extensively in IEEE/ACM Transactions on Audio, Speech and Language Processing, ICASSP, and Interspeech.
Speech for Healthcare: His recent work on spoken language biomarker selection enables medical professionals to determine the most discriminative biomarkers for speech-based dementia detection. He also pioneers the disentanglement of speaker features for speech-based depression detection.
Bioinformatics and Biomedical Engineering: Professor Mak has extensive contributions in bioinformatics and biomedical engineering. His research papers have appeared in Journal of Theoretical Biology, Bioinformatics, BMC Bioinformatics, PLoS One, IEEE Journal of Biomedical and Health Informatics, IEEE/ACM Trans. on Computational Biology and Bioinformatics, among other renowned journals in the field with very rigorous criteria for publication. Professor Mak has also co-authored a book, Machine Learning for Protein Subcellular Localization Prediction. Professor Mak is the first researcher who brought the idea of i-vectors to the biomedical engineering community. In particular, he applied i-vectors to capture the characteristics of patient-dependent heartbeats and used the i-vectors to adapt a patient-independent ECG classifier to patient dependent one.
We use Cookies to give you a better experience on our website. By continuing to browse the site without changing your privacy settings, you are consenting to our use of Cookies. For more information, please see our Privacy Policy Statement.
Your browser is not the latest version. If you continue to browse our website, Some pages may not function properly.
You are recommended to upgrade to a newer version or switch to a different browser. A list of the web browsers that we support can be found here