AMA 50th Anniversary young Alumni Greater Bay Area Research workshop
Conference / Workshop
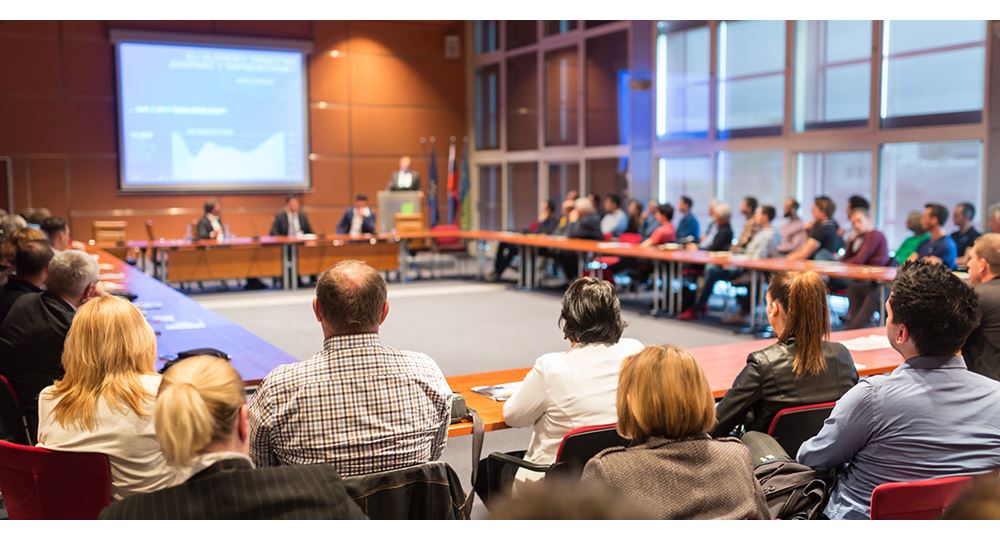
-
Date
01 Nov 2022
-
Organiser
Dr. Binyan Jiang, The Hong Kong Polytechnic University
-
Time
14:30 - 17:30
-
Venue
Online Talk via Zoom
Speaker
Dr. Yaohua Hu, College of Mathematics and Statistics, Shenzhen University
Dr. Hai-Yang Jin, South China University of Technology
Dr Lei Yang, School of Computer Science and Engineering at Sun Yat-Sen University
Summary
Keynote Speaker
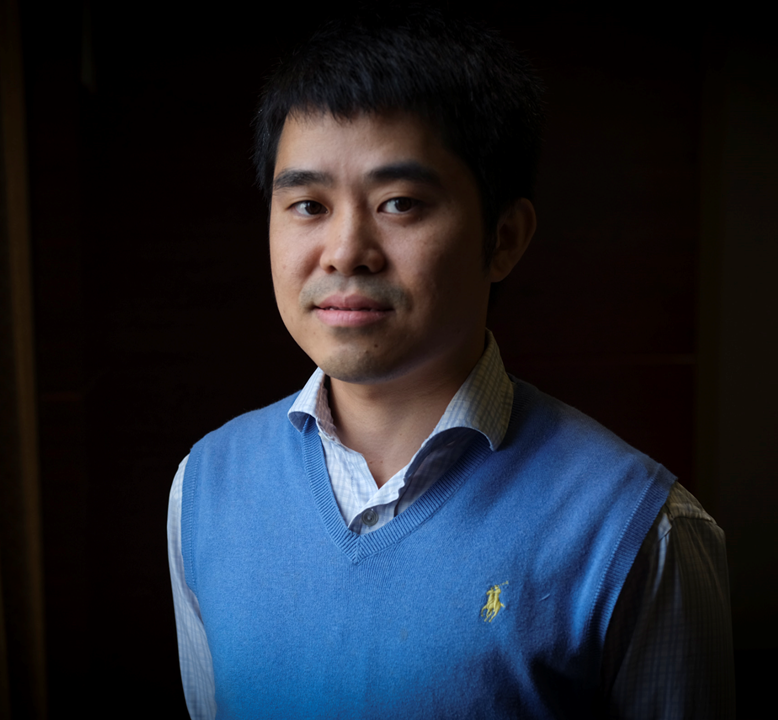
Dr. Yaohua Hu, College of Mathematics and Statistics, Shenzhen University
Dr. Yaohua Hu received his B.S. in Mathematics (Chu Kechen Honors College) and M.S. in Computational Mathematics from Zhejiang University in 2007 and 2009, respectively, and Ph.D. in Applied Mathematics from Hong Kong Polytechnic University (supervised by Prof. Xiaoqi YANG) in 2013. Then he continued his research work in Hong Kong Polytechnic University and Zhejiang University as a postdoctoral fellow. Yaohua Hu joined College of Mathematics and Statistics at Shenzhen University in May 2015, and now he is an associate professor there. His current research interests include theory and algorithms for large-scale optimization, and their various applications in statistics, machine learning, bioinformatics, and image processing.
Awards:
- The National Science Foundation of China for Outstanding Young Scholars, 2022 (國家優青)
- First Prize of the outstanding research output Award of Computational Mathematic, 2021
- Shenzhen University “荔園優青”,2020 年;
- High-Level Talent in Nanshan District of Shenzhen, Shenzhen, March 2017.
- First Prize of the Best Paper Award of Computational Mathematics, Guangdong, November 2016.
- Overseas High-Caliber Personnel, Shenzhen, August 2015.
Talk information:
Sparse Optimization and Gene Regulatory Networks
Abstract:
Sparse optimization, aiming to find sparse solutions of linear inverse problems, is a popular research topic in applied mathematics and optimization, and nonconvex sparse optimization methods have been shown to enjoy efficient and robust sparsity promotion capability in vast applications. Gene regulatory networks is a fundamental tool in systems biology and biochemistry, while inferring gene regulatory networks from gene expression data at whole genome level is an arduous challenge.
In this talk, we will build up a bridge between sparse optimization and gene regulatory networks, and propose several nonconvex sparse optimization models for gene regulatory networks with different structures. Moreover, we will present the mathematical theory for nonconvex sparse optimization problems, including the consistency theory, the asymptotic theory and the stability theory of the model and the convergence theory of first-order iterative methods
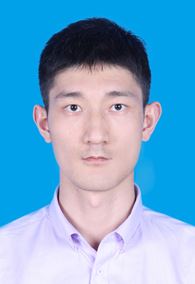
Dr. Hai-Yang Jin, South China University of Technology
Dr. Haiyang Jin received his B.Sc (2009) at Central China Normal University, M.Sc degrees (2011) in Mathematics at Wuhan University, and PhD (2014) in the Department of Applied Mathematics at the Hong Kong Polytechnic University under supervisions of Prof. Zhi-An Wang. He is currently a Professor in the School of Mathematics, South China University of Technology.
Awards:
Excellent Youth Foundation of GuangDong province (廣東省傑青)
Talk information:
Global dynamics and spatio-temporal patterns of predator-prey systems with taxis mechanism
Abstract:
Compared with the existing well-known results on the ODE type predator-prey systems, few results are known for the predator-prey systems with taxis mechanism. In this talk, we first establish the existence of global classical solution for some predator-prey models with various type of taxis (e.g. prey-taxis and alarm-taxis ) by using the appropriately respect the particular structure and energy estimates. Moreover, by constructing Lyapunov functionals, we also establish the global stability of the spatially homogeneous steady state under certain conditions. With numerical simulations, we further demonstrate that spatio-temporal patterns are possible for the parameters outside the stability regime.
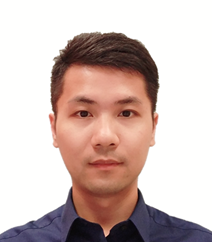
Dr Lei Yang, School of Computer Science and Engineering at Sun Yat-Sen University
Dr Yang received his B.Sc (2011) and M.Sc degrees (2014) in Applied Mathematics at Tianjin University, and PhD (2017) in the Department of Applied Mathematics at the Hong Kong Polytechnic University under supervisions of Prof. Xiaojun Chen (chief-supervisor) and Prof. Ting Kei Pong (co-supervisor). He is now a tenure-track associate professor in School of Computer Science and Engineering at Sun Yat-Sen University. His research interests include Efficient methods for large-scale (convex or nonconvex) optimization problems in data science, and Computational optimal transport.
Awards:
中山大學百人計劃
Hong Kong Mathematical Society Best Thesis Award, 2019
SIAM Certificate Award, 2017
Talk Information:
Bregman Proximal Point Algorithm Revisited: A New Inexact Version and its Inertial Variant
Joint work with Kim-Chuan Toh
Abstract:
In this talk, we focus on a general convex optimization problem, which covers various classic problems in different areas and particularly includes many optimal transport related problems arising in recent years. To solve this problem, we revisit the classic Bregman proximal point algorithm (BPPA) and introduce a new inexact stopping condition for solving the subproblems, which can circumvent the underlying feasibility difficulty often appearing in existing inexact conditions when the problem has a complex feasible set. Our inexact condition also covers several existing inexact conditions as special cases and hence makes our inexact BPPA (iBPPA) more flexible to fit different scenarios in practice. As an application to the standard optimal transport (OT) problem, our iBPPA with the entropic proximal term can bypass some numerical instability issues that usually plague the popular Sinkhorn's algorithm in the OT community. The iteration complexity of $O(1/k)$ and the convergence of the sequence are also established for our iBPPA under some mild conditions. Moreover, inspired by Nesterov's acceleration technique, we develop an inertial variant of our iBPPA, denoted by V-iBPPA, and establish the iteration complexity of $O(1/k^{\lambda})$, where $\lambda\geq1$ is a quadrangle scaling exponent of the kernel function. In particular, when the proximal parameter is a constant and the kernel function is strongly convex with Lipschitz continuous gradient (hence $\lambda=2$), our V-iBPPA achieves a faster rate of $O(1/k^2)$ just as existing accelerated inexact proximal point algorithms. Some preliminary numerical experiments for solving the standard OT problem are conducted to show the convergence behaviors of our iBPPA and V-iBPPA under different inexactness settings. The experiments also empirically verify the potential of our V-iBPPA for improving the convergence speed.