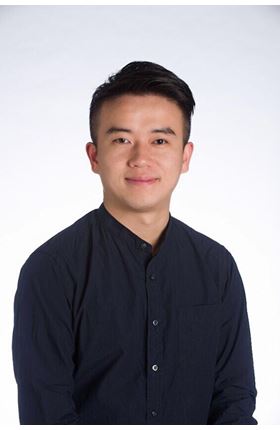
Biography
Prof Cheng received his Ph.D. degree from the Department of Mechanical Engineering of the University of Melbourne. He was appointed a Visiting Researcher in the Department of Mechanical Engineering of the University of California, Berkeley from 2017 to 2018, and a Postdoc in the Energy Systems Division of Argonne National Laboratory from 2019 to 2021. In 2021, Prof Cheng joined the Department of Mechanical Engineering of the Hong Kong Polytechnic University as an Assistant Professor.
Prof Cheng’s current research interests include gas-phase chemical kinetics, trans-/super-critical combustion fundamentals, uncertainty quantification, and near real-time model optimization. He is particularly interested in integration of green fuels and propellants in heavy-duty engines, gas turbines and next-generation space applications, via generating new understandings and knowledge of intermolecularly interactive chemical kinetics. He is also specifically interested in characterizing kinetics-governed phenomena under extreme conditions using both theoretical and experimental techniques, with a particular emphasis on trans-/super-critical pyrolysis, oxidation and combustion. He also has special focus on developing machine learning assisted frameworks to implement near real-time optimization of high-dimensional models, as well as to enable detailed chemical kinetics in turbulent combustion modeling.
Applications with proven track record and strong commitment are kindly invited for Ph.D., RA and other research openings in Prof Cheng’s group.
Education and Academic Qualifications
- Doctor of Philosophy, University of Melbourne