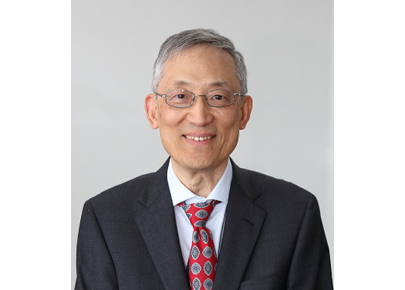
Prof. Yin Zhang
The Chinese University of Hong Kong, Shenzhen
Time: 09:00 - 09:40
Title:
Introduction to Practical Interior-Point Methods
Abstract:
Primal-dual interior-point methodology is a general-purpose formulation for accurately, reliably and efficiently solving many classes of "inequality" constrained optimization problems. We motivate this methodology from the perspective of effectively applying Newton's method. We first focus on linear programming, then extend to conic and nonlinear programming. We will use an example to demonstrate how to apply this methodology to effectively solving structured problems. The presentation is accessible for non-expert audience members such as graduate students who have acquired a basic knowledge on optimization.
Biography
Prof. Yin Zhang is currently a Presidential Chair professor in the School of Data Science at The Chinese University of Hong Kong, Shenzhen. He received a PhD degree in Applied Mathematics from SUNY at Stony Brook in 1987. He was a postdoctoral fellow at Rice University and an assistant professor at the University of Maryland Baltimore County. From 1996 to 2021, he worked as an associate and then full professor in the Department of Computational and Applied Mathematics at Rice University. His research focuses on optimization algorithms and applications. He is a SIAM Fellow and served on various editorial boards of journals in the areas of optimization and computational mathematics, including SIAM Journal on Optimization and Mathematical Programming Computation.
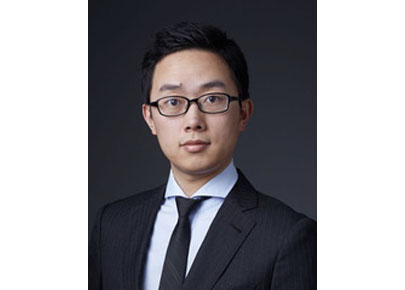
Prof. Qian Hu
Nanjing University
Time: 09:40 - 10:20
Title:
Branch-and-price for Two-dimensional Vector Packing Problems
Abstract:
Vector packing problems and its variants have important applications in many areas. Such a problem is to pack a set of different items into some bins without violating a vector of capacities. The objective is to minimize the number of bins or the total cost. In some application in transportation and logistics, the volumetric weight of a package is considered to be an essential factor in calculating the delivery cost of shipments. In this talk, we will discuss some exact algorithms for the two-dimensional vector packing problem and some of its variants. The exact algorithms solve a set-partitioning formulation involving a large number of columns. Column generation is thus utilized and the exact algorithms are so-called branch-and-price algorithms. We show the algorithm design on solving the pricing problems to produce the necessary columns and the techniques for accelerating the exact algorithms.
Biography
Qian Hu is an associate professor of the School of Management and Engineering, Nanjing University. He received a PhD degree in management science from City University of Hong Kong. Qian Hu’s research is mainly on heuristic and exact algorithms for combinatorial optimization and integer programming problems, and applications in packing, routing, transportation and scheduling. His works have been published on the journals such as European Journal of Operational Research、Transportation Science、Transportation Research Part B, C.
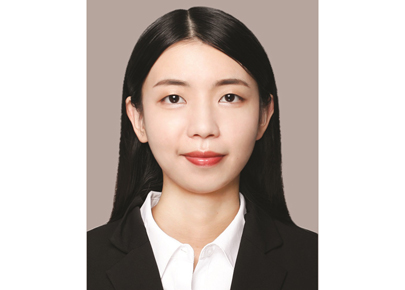
Dr Junyan Liu
Huawei
Time: 10:30 - 11:10
Title:
Several Challenging Integer Linear Programming Problems in Practical Optical Networks
Abstract:
With the advent of 5G era, the traffics demand in optical networks has increased dramatically. To accommodate such huge traffics, efficient resources allocation and topology design in optical networks are needed. In this talk, we will introduce the basic resources allocation problem in optical network, the routing and wavelength assignment(RWA) problem, and some topology design problems, including regenerator placement and traffic grooming problem. In practice, the fiber may break down. Thus, the survivable resources allocation and topology design with protection are also included.
Biography
Junyan Liu is an optimization researcher in Hong Kong Theory Lab, Huawei Hong Kong Research Center. She received the B.S. degree in electronics and information engineering from Huazhong University of Science and Technology (HUST) in 2015, and the Ph.D. degree in electronic and computer engineering from Hong Kong University of Science and Technology (HKUST), in 2020. Her current research interests include large-scale optimization techniques with applications in networks problems.
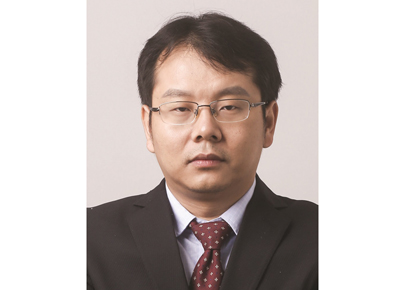
Prof. Hu Qin
Huazhong University of Science and Technology
Time: 11:10 - 11:50
Title:
The Pickup and Delivery Problem with Time Windows and Incompatibility Constraints in Cold Chain Transportation
Abstract:
This study investigates a new variant of the pickup and delivery problem with time windows (PDPTW) applied in cold chain transportation, which quantifies the effect of time on the quality of perishable products. Multiple commodities with incompatibility constraints are considered, where some types of products cannot be transported in a vehicle simultaneously due to their different properties and requirements for storage temperatures. The aim is to determine vehicles’ pickup and delivery routes as well as their departure times from
the depot such that the travel cost and refrigeration cost of vehicles and the quality decay cost of products are minimized. We formulate this problem as a set partitioning model, which is solved exactly by a tailored
branch-and-price (B&P) algorithm. To tackle the asymmetry issue arising from the pricing problem of the B&P framework, we develop a novel asymmetric bidirectional labeling algorithm. Benchmark instance sets based on real-world statistical data and classic PDPTW instance sets are first generated for this problem. Numerical results show that our B&P algorithm can solve most instances to optimality in an acceptable time frame. Moreover, our results demonstrate that integrating the refrigeration and quality decay costs into the objective
function can significantly lower the total cost of cold chain transportation activities, compared to the widely adopted objective function minimizing only the travel cost.
Biography
Hu Qin received the Ph.D. degree from the City University of Hong Kong, Hong
Kong, in 2011. He is currently a Professor with the School of Management, Huazhong
University of Science and Technology. His current research interests are in the fields of
algorithms and artificial intelligence, including various topics in operations research,
such as vehicle routing problem, freight allocation problem, container loading problems, and transportation problems.
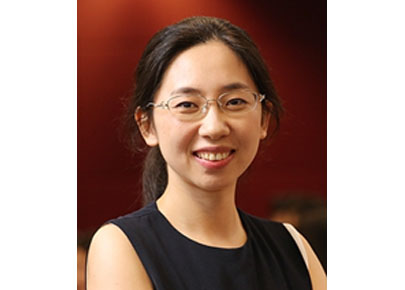
Dr Jin Qi
The Hong Kong University of Science and Technology
Time: 11:50 - 12:30
Title:
Supermodularity in Two-stage Distributionally Robust Optimization
Abstract:
In this talk, we solve a class of two-stage distributionally robust optimization problems which have the property of supermodularity. We exploit the explicit worst-case expectation of supermodular functions and derive the worst-case distribution for the robust counterpart. This enables us to develop an efficient method to obtain an exact optimal solution to these two-stage problems. Further, we provide a necessary and sufficient condition for checking whether any given two-stage optimization problem has the supermodularity property. We also investigate the optimality of the segregated affine decision rules when problems have the property of supermodularity. We apply this framework to several classic problems, including the multi-item newsvendor problem, the facility location problem, the lot-sizing problem on a network, the appointment scheduling problem, and the assemble-to-order problem. While these problems are typically computationally challenging, they can be solved efficiently under our assumptions. Finally, numerical examples are conducted to illustrate the effectiveness of our approach.
Biography
Jin Qi is currently an associate professor in the Department of Industrial Engineering and Decision Analytics at the Hong Kong University of Science and Technology. She received her PhD degree in the NUS Business School, National University of Singapore. Her research interest lies in the distributionally robust optimization with its applications in operations management, including healthcare and transportation.
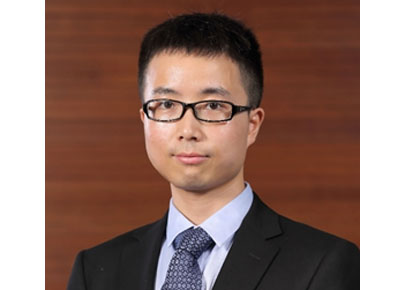
Dr Kai Pan
The Hong Kong Polytechnic University
Time: 14:30 - 15:10
Title:
Computationally Efficient Approximations for Distributionally Robust Optimization under Moment and Wasserstein Ambiguity
Abstract:
Distributionally robust optimization (DRO) is a modeling framework in decision-making under uncertainty where the probability distribution of a random parameter is unknown while its partial information (e.g., statistical properties) is available. In this framework, the unknown probability distribution is assumed to lie in an ambiguity set consisting of all distributions compatible with the available partial information. Although DRO bridges the gap between stochastic programming and robust optimization, one of its limitations is that its models for large-scale problems can be significantly difficult to solve, especially when the uncertainty is highly-dimensional. In this talk, we propose computationally efficient inner and outer approximations for DRO problems under a piece-wise linear objective function and with a moment-based ambiguity set and a combined ambiguity set including Wasserstein distance and moment information. In these approximations, we split a random vector into smaller pieces, leading to smaller matrix constraints. In addition, we use principal component analysis to shrink uncertainty space dimensionality. We quantify the quality of the developed approximations by deriving theoretical bounds on their optimality gap. We display the practical applicability of the proposed approximations in a production-transportation problem and a multi-product newsvendor problem. The results demonstrate that these approximations dramatically reduce the computational time while maintaining high solution quality. The approximations also help construct an interval that is tight for most cases and includes the (unknown) optimal value for a large-scale DRO problem, which usually cannot be solved to optimality (or even feasibility in most cases).
Biography
Dr Kai Pan is currently an Associate Professor in the Department of Logistics and Maritime Studies at the Faculty of Business of The Hong Kong Polytechnic University(PolyU) and the Director of the MSc Program in Operations Management (MScOM). He received his Ph.D. and M.S. degrees from the University of Florida, USA, and his Bachelor's degree from Zhejiang University, China. Previously he worked as a Research Scientist at Amazon (Seattle, Washington) on Supply Chain Optimization and a Power System Engineer at GE Grid Solutions (Redmond, Washington) on Electricity Market Operations. His research interests include Stochastic and Discrete Optimization, Robust and Data-Driven Optimization, Dynamic Programming, and their applications in Energy Market, Smart City, Supply Chain, Shared Mobility, Marketing, and Transportation. His research on these topics has been published in Operations Research, INFORMS Journal on Computing, Production and Operations Management, IISE Transactions, European Journal of Operational Research, IEEE Transactions on Power Systems, Transportation Research Part B, etc. Dr Pan was the first-place winner of the prestigious IISE Pritsker Doctoral Dissertation Award in 2017. His works have been supported by the Research Grants Council (RGC) of Hong Kong and the National Natural Science Foundation of China.
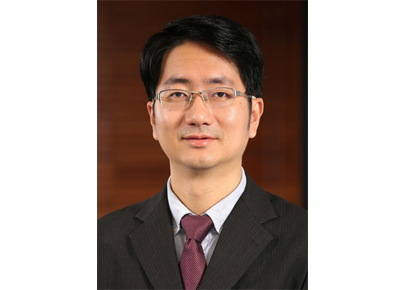
Prof. Zhou Xu
The Hong Kong Polytechnic University
Time: 15:10 - 15:50
Title:
A Dynamic Discretization Discovery Algorithm for Continuous-Time Service Network Design with Holding Costs
Abstract:
In this talk, we present a new dynamic discretization discovery algorithm to tackle the continuous-time service network design problem (CTSNDP) in freight transportation with holding costs considered. The CTSNDP occurs widely in practice, and it aims to minimize the total operational cost for consolidation carriers by optimizing schedules of transportation services and routes of shipments for dispatching along a continuous-time planning horizon. To be cost effective, shipments often wait to be consolidated, which incurs holding costs. Despite its importance, such holding costs have not been considered in existing exact solution methods for the CTSNDP, since incorporating it significantly complicates the problem. By tackling this challenge, our newly developed dynamic discretization discovery algorithm can solve the CTSNDP with holding cost to exactly optimum. It is based on a novel relaxation model, a new upper bound heuristic, and a new discretization refinement procedure. Results of our computational experiments demonstrate its effectiveness and the efficiency in both finding optimal solutions and producing tight lower and upper bounds.
Biography
Zhou XU is currently a Professor of the Department of Logistics and Maritime Studies in the Faculty of Business at the Hong Kong Polytechnic University. He obtained his Ph.D. degree from the Hong Kong University of Science and Technology, master’s degree from the National University of Singapore, and bachelor’s degree from Tsinghua University. His research focuses on operations research and algorithm design as well as their applications to various challenging optimization problems, for helping make better decisions. His publications mainly appear in premier journals in operations research and transportation, such as Operations Research, INFORMS Journal on Computing, Transportation Science, Transportation Research Part B, and so on.
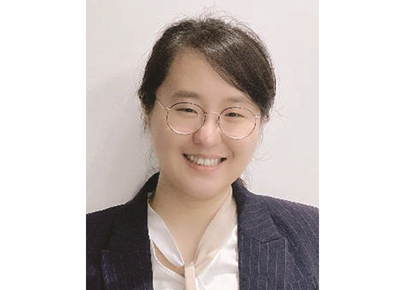
Dr Hui-Ling Zhen
Noah's Ark Lab, Huawei
Time: 16:00 - 16:40
Title:
Heuristic Search for Combinatorial Optimization in EDA
Abstract:
Boolean satisfactory (SAT) plays an important role in EDA, including but not limit to logic synthesis, logic equivalence checking (LEC) and automatic test pattern generation (ATPG). The core idea of SAT is CDCL, which is a conflict-driven heuristic search from the perspective of combinatorial optimization. In this talk, we aim to show some analysis for the application of SAT in EDA, from experience and lessons. Meanwhile, we also aim to show some learning-aided methods in industrial scenarios.
Biography
Hui-Ling Zhen is a research scientist in Huawei, Hong Kong. Before that, she is a post-doctoral research fellow in City University of Hong Kong, after she received the B.S. degree in numerical mathematics and the Ph.D. degree in applied mathematics. Her current research interests include large-scale optimization, constraint programming, as well as their applications in industrial verification and testing.
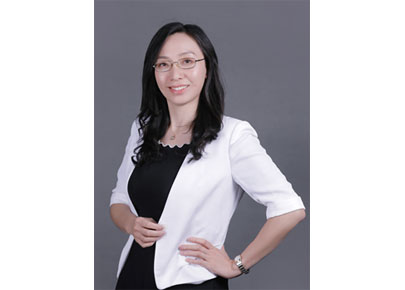
Prof. Jie Song
Peking University
Time: 16:40 - 17:20
Title:
Bandits with Correlated Contexts: Application to Product Ranking under Evolving Consumer Review
Abstract:
In this paper, we study a formulation of contextual bandits where the contexts might be arbitrarily correlated over time. We are motivated by ranking problems in e-commerce platforms, where consumers leave reviews about their experiences with products or services. The bodies of reviews grow over time, providing valuable sources of information. Subsequent consumers as well as the platforms, make use of the information to learn about the quality of the products or services. The platforms, in particular, use the information to better rank the options available to consumers in order to improve consumer satisfaction or to improve sales. We study the performance of an online learning algorithm under time-correlated contexts. We benchmark our algorithm against one that has access to the ground truth. To do this, we show how to perform estimation with correlated and non-identically distributed observations, and we derive a novel finite-sample result for maximum likelihood estimators for samples that are neither independent nor identically distributed. Although the algorithm is customized to our problem of interest, which is a personalized ranking problem, it can be easily adapted to other problems. One major insight we cover is that there is an operationally relevant distinction between perceived quality and true quality. Perceived quality influences demand for a product or service, whereas true quality measures customers’ ultimate satisfaction with a product. Estimates of true quality from reviews tend to be inflated and likely do not converge over time due to selection bias. However, even though it might not be possible to obtain unbiased estimates of true quality, it is still possible to design algorithms to rank that achieve diminishing regret at a non-trivial rate.
Biography
Dr Jie Song is a professor in Department of Industrial Engineering and Management and the Associate Dean with the College of Engineering of Peking University. She has been awarded Chang Jiang Professor by the Ministry of Education. She received the B.S. degree in Applied Mathematics from Peking University, Beijing, China, in 2004, and the Ph.D. degree in Department of Industrial Engineering from Tsinghua University, Beijing, in 2010. She was a postdoctoral associate at University of Madison, and Visiting Scholar in George Institute of Technology and Columbia University. Her research interests include stochastic simulation and optimization, and online algorithm design, with applications in resource allocation of complex service systems. Her research contributes to the major government strategic planning, and provide scientific quantitative decisions. Her research has been funded by National Natural Science Foundation of China (NSFC) and Chinese Ministry of Science and Technology. She is the associate editor of leading journals, such as IEEE Transaction on Automation Science and Engineering, and serves as the co-chair of Automation Management Technical Committee of IEEE Robotics and Automation Society (RAS). She won the Best Paper Award from RAS at IEEE CASE in 2013 and IEEE TASE in 2020, also the Honorable Mention Award by IISE in 2020 and 2021. She is the recipient of Teaching Excellence Award of Peking University in 2016 and 2017.
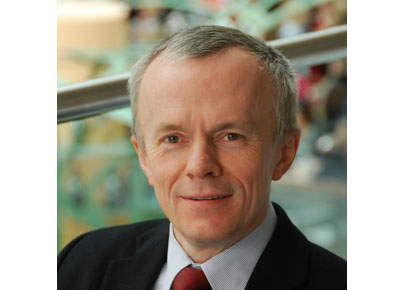
Prof. Jacek Gondzio
University of Edinburgh
Time: 17:20 - 18:00
Title:
Applications of Interior Point Methods: from Sparse Approximations to Discrete Optimal Transport
Abstract:
A variety of problems in modern applications of optimization
require a selection of a 'sparse' solution, a vector with
preferably few nonzero entries. Such problems may originate
from very different applications in computational statistics,
signal or image processing or compressed sensing, finance,
machine learning and discrete optimal transport, to mention
just a few. Sparse approximation problems are often solved
with dedicated and highly specialised first-order methods
of optimization. In this talk I will argue that these problems may be very
efficiently solved by the more reliable optimization techniques
which involve some use of the (inexact) second-order information
as long as this is combined with appropriately chosen iterative
techniques of linear algebra, such as for example methods
from the Krylov-subspace family. Two particular classes of methods, the Newton Conjugate Gradient
and the Interior Point Method will be interpreted as suitable homotopy
type approaches and will be applied to solve problems arising from:
compressed sensing, multi-period portfolio optimization,
classification of data coming from functional Magnetic Resonance Imaging,
restoration of images corrupted by Poisson noise,
classification via regularized logistic regression, and
discrete optimal transport. In all these cases, the performance of the proposed methods
will be compared against competitive first-order methods.
Computational evidence will be provided to demonstrate
that specialized second-order methods compare favourably
and often outperform the cutting-edge first-order methods.
Biography
Professor Jacek Gondzio holds an engineering degree (1983)
and a PhD in Electronics (1989) both from the Department
of Electronics, Warsaw University of Technology.
Since October, 1998 he has been at the School of Mathematics,
the University of Edinburgh. His research interests include the theory and implementation
of algorithms for very large-scale optmization. He is best known
for his contributions in the area of interior point methods.
His joint work with Andreas Grothey led to a development of the
Object Oriented Parallel Solver (OOPS) which was applied in 2005
to solve a financial planning problem with more than a billion
decision variables, a world record then.
His more recent works focused on solving various L1-regularized
sparse approximation problems originating from computational
statistics, signal or image processing or compressed sensing,
finance, machine learning and discrete optimal transport,
to mention just a few. He is a member of Editorial Board of several leading optimization
journals: Computational Optimization and Applications,
Mathematical Programming Computation,
Optimization Methods and Software, and
European Journal of Operational Research.